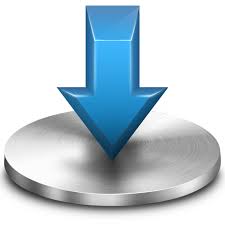

Moreover, a 19% reduction in the RMSE is obtained using our approach in comparison to existing supervised learning models.", Also, our approach leads to accurate RUL estimates with a Root Mean Square Error (RMSE) of 2.67 flights only. We show that the consideration of the operating conditions improves the monotonicity of the health indicators by 97%. We illustrate our approach for turbofan engines. These health indicators are further used to predict the RUL of the aircraft system using a similarity-based matching approach. We show that the consideration of the operating conditions leads to robust health indicators and improves significantly the monotonicity, trendability and prognosability of these indicators. Since aircraft fly under various operating conditions (varying altitude, speed, etc.), these conditions are also integrated in the autoencoder. This autoencoder is trained with unlabelled data samples (i.e., the true RUL is unknown). We therefore propose a Long Short-Term Memory (LSTM) autoencoder with attention to develop health indicators for an aircraft system instead. There are thus very few labelled data samples available. In aviation, however, aircraft systems are often preventively replaced before failure. Moreover, a 19% reduction in the RMSE is obtained using our approach in comparison to existing supervised learning models.Ībstract = "Most Remaining Useful Life (RUL) prognostics are obtained using supervised learning models trained with many labelled data samples (i.e., the true RUL is known).

Most Remaining Useful Life (RUL) prognostics are obtained using supervised learning models trained with many labelled data samples (i.e., the true RUL is known).
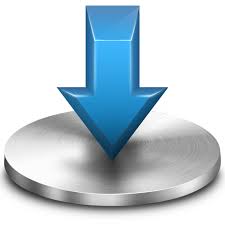